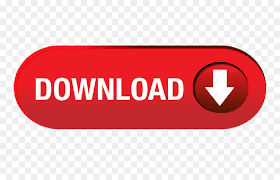

- We need to go deeper platforms software#
- We need to go deeper platforms code#
- We need to go deeper platforms series#
NielsenNet: A Guided ExampleĪs a simple example of how Slim builds more complicated architectures, consider the MNIST classifier that is presented in Chapter 6 of Michael Nielsen’s wonderful textbook “Neural Networks and Deep Learning.” The neural network, which I’ve christened “NielsenNet” consists of: Even more conveniently, Slim does all of this under a named scope that you provide allowing you to navigate your architecture in Tensorboard. Slim will do all of the heavy lifting it defines the appropriate weight and bias variables and links them in the appropriate way.
We need to go deeper platforms code#
For instance, the following code will create a neural network with two layers, each with 256 hidden units: def my_neural_network(input): net = slim.fully_connected(input, 256, scope='layer1-256-fc') net = slim.fully_connected(net, 256, scope='layer2-256-fc') return net input = load_data() output = my_neural_network(input) Using slim makes it simple to chain multiple building blocks together. Access it by simply importing it: import as slim Slim is Tensorflow library that bundles commonly used building blocks like convolution and max pooling. However, as the complexity of our architecture grows, these simple primitives become cumbersome. Tensorflow provides many primitives for defining these graphs and if you’ve run through the introductory tutorials you’ve undoubtedly encountered them when constructing simple neural net architectures. Defining an architecture for a learning task is tantamount to defining this graph.

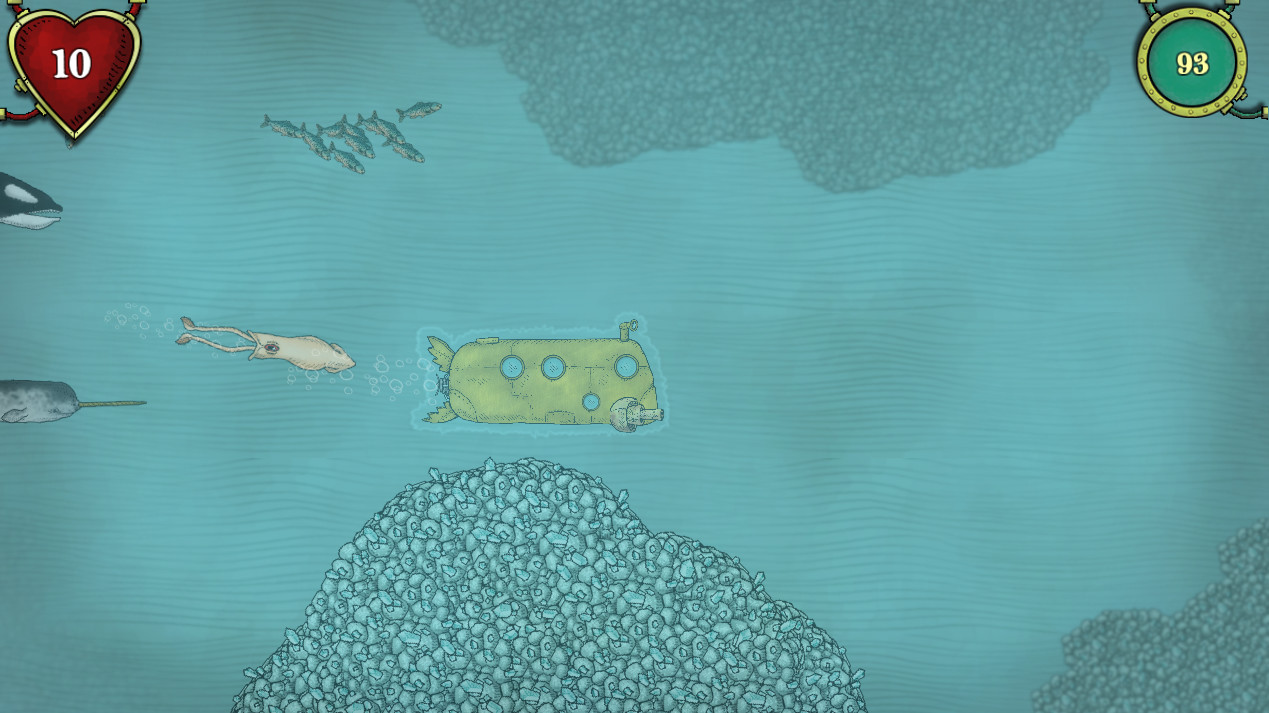
Operations in our neural network (e.g., convolution, bias adding, dropout, etc.) are all modeled as nodes and edges in this graph. Check it out here.Īt the core of Tensorflow is the notion of a computational graph. I’ve also created a Github repository with code samples that we’ll use in the series. Signup is fast and easy and gives you access to a desktop computing environment through your browser.
We need to go deeper platforms software#
Think graduate student embarking on a project, or a software engineer who’s been asked to build a training and inference pipeline. Instead, this tutorial is aimed at folks who have done the basic Tensorflow tutorials and want to “go a little deeper” to apply Inception to their own projects. This is a practical introduction, so it’s not focused on the theories that underly neural networks, computer vision, or deep learning models (though there will be a few remarks about the general motivation behind Inception in Part 2). While the goal isn’t to get to world-class performance, we’ll get a model that performs at >99% accuracy. Inspired by Inception’s own origins, this tutorial will “go deeper,” presenting a soup-to-nuts tutorial using Inception to train a MNIST (hand-written digits) classifier.

We need to go deeper platforms series#
I wrote this series because I couldn’t easily bridge the gap between Tensorflow’s tutorials and doing something practical with Inception. It’s also designed to be computationally efficient, using 12x fewer parameters than other competitors, allowing Inception to be used on less-powerful systems. The Inception model is particularly exciting because it’s been battle-tested, delivering world-class results in the widely-acknowledged ImageNet Large Scale Visual Recognition Challenge (ILSVRC). When Google released its Tensorflow framework and Inception architecture, I decided to do a deep dive into both technologies in my spare time. I’ve nursed a side interest in machine learning and computer vision since my time in graduate school. We Need to Go Deeper: A Practical Guide to Tensorflow and Inception
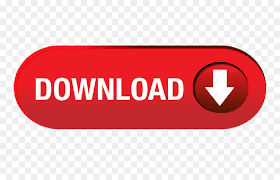